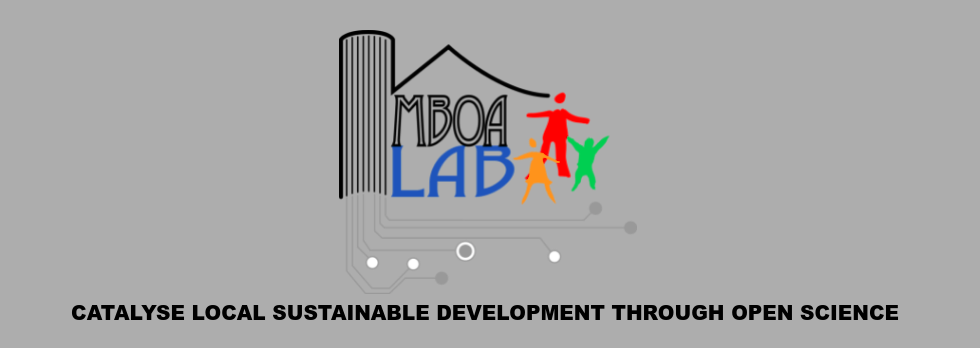
MboaLab is an open and collaborative space located in Yaoundé, Cameroon. The aim of MboaLab is to catalyse sustainable local development and improve people’s living conditions through open science.
Aim and missions
- provide community and lifelong education for the population, as well as formal education for the younger generations
- serve as a platform for exchanges on issues related to local context
- propose solutions that meet the needs of communities, using local knowledge and open digital technologies
- raise public awareness of environmental issues
- facilitate access to basic health care
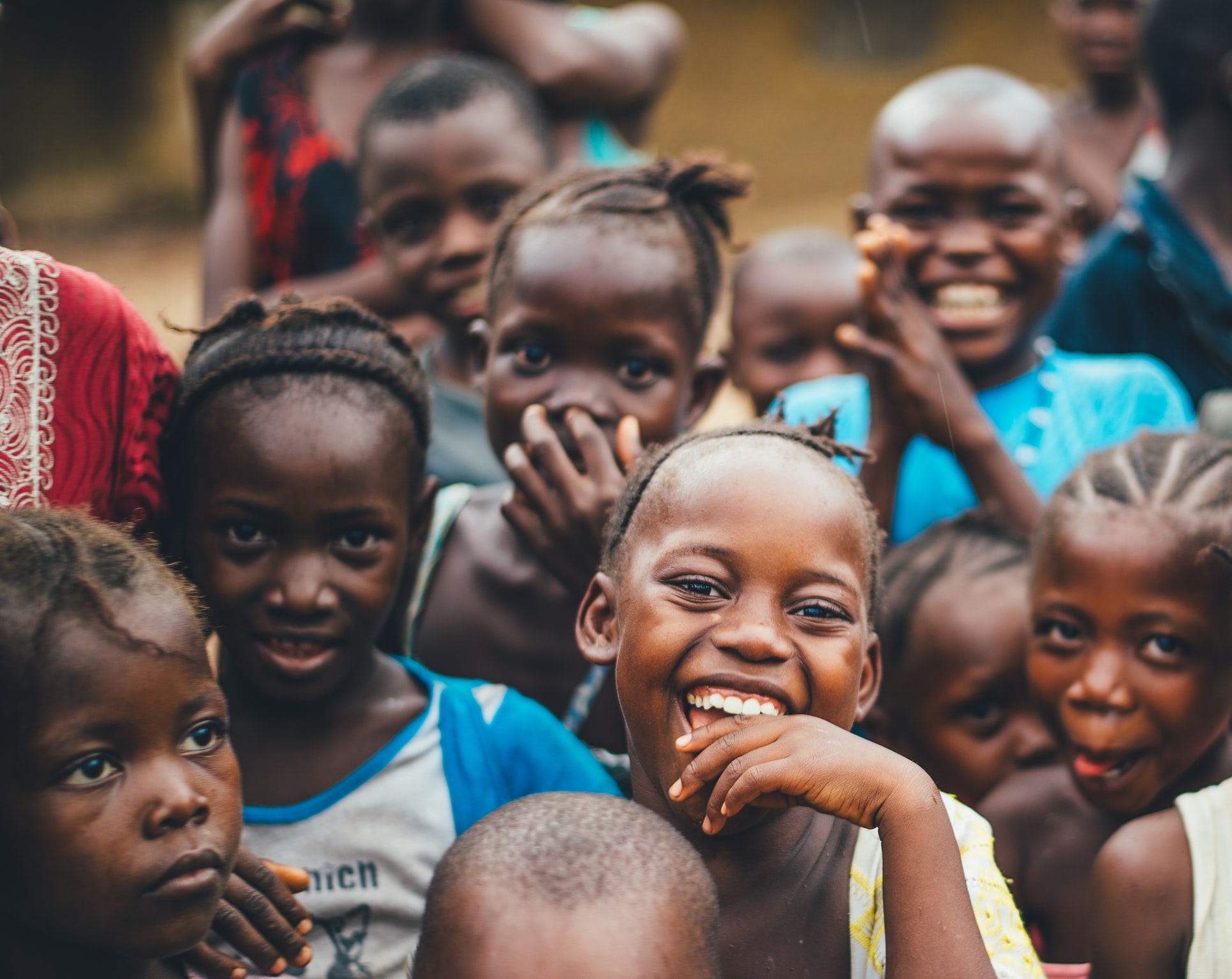
Open Projects
Project Description
Improve diagnostics of typhoid through Open Science: An Artificial Intelligence-based technique
ContextTyphoid or Enteric fever is one of the infectious human diseases in Cameroon and Africa. Outbreaks of typhoid fever caused by Salmonella typhi remains a serious health problem worldwide. There are a number of tests available presently, from molecular to immunological and biochemical to microbiological. Some well-known and conventional Method of Diagnosis of Typhoid Fever are :
So, misdiagnosis is usually experienced since most health care facilities use only Widal test without confirmation of results with a second test method. In addition, the diagnosis of Typhoid involves several levels of uncertainties. Patients cannot tell exactly how they feel, doctors and nurses cannot tell exactly what they observe.
Opportunities and solutionAugmented intelligence makes more sense than artificial intelligence, especially in tropical diseases such as Typhoid. This is because it highlights the enhanced capabilities of a human when augmented with the right tools and technologies.In a sensitive industry such as healthcare, human intelligence cannot be replaced. Augmented intelligence specifies systems that augment human intelligence rather than attempt to replace them. Combining AI systems with an irreplaceable human clinician can advance better diagnosis. We propose to use the microcultures test with the blood which seems to be the best accepted by the laboratory technicians. We will use CNNs as algorithms on the collected images to train the algorithm. Everything will depend on the volume of images we have, if we have few we will use transfer learning to automate the test of microbiological cultures .As we saw above this test is reliable at 61% and to reinforce the reliability of this test we will use the second algorithm which is a fast decision tree learner. This algorithm based on 18 symptoma variables will allow us not only to confirm the diagnosis but above all to determine the level of severity of the disease. The main challenge our project is facing is availability of local and high quality open data.